
Everyone and their cousin is trying to harness the power of AI these days. It’s hard to miss the constant flow of articles, social media posts, or Elon Musk’s dark prophecies about artificial intelligence. And this is not just in the tech space. The language of AI has entered into the mainstream of conversations across all industries.
In consumer lending, logistic regression has been the gold standard for decades in predicting who is going to default on a loan. It now appears on the verge of losing its place on top of the podium, with AI promising to push the boundaries of predictability even further: faster and more informed decisions, real-time fraud monitoring, way better user experience — this is just a start. For many companies, the race is on.
But here’s the thing: we cannot develop AI solutions in a vacuum — you need to know exactly what problems you are trying to solve. And I mean all the problems, not just how predictive a solution is. If we do not carefully think about how to implement a risk strategy using the power of AI to account for issues such as explainability, regulation, and fairness, then we run the risk of spending a lot of money, wasting a lot of time, and in the end, incorrectly concluding that our lending practices would not benefit from AI.
Here’s the thing: we cannot develop AI solutions in a vacuum — you need to know exactly what problems you are trying to solve.
I have devoted my career to data science and the pursuit of analytical solutions to solve problems in consumer lending. I have been on both sides of the issue, leading data science and risk management teams alike. In this series of articles, I intend to share insights and practical guidance to help you with your own AI journey. Let’s jump in!
The solution in search of a problem
Overheard in an office near you:
“We must have an AI strategy.”
“Our competitors are using AI — so should we.”
“We are the best AI company! Hire us, and we will solve all of your problems.”
Right? We’ve all heard variations of these statements. It’s part of the zeitgeist: in order to stay competitive, we must be using AI. Right now, banks are spending billions exploring and setting up sophisticated data science teams in the hopes that their AI strategy will provide a competitive advantage.
We know there is a buzz — but with so many unknowns, AI quickly feels like a solution in search of a problem.
As credit risk management professionals, we often become nervous when we’re put in front of a “new and improved” way of doing things. It happened before when credit scoring first appeared in 1958. And it’s happening again when an internal data science team or an AI vendor says we should be using AI to develop credit and fraud models. We know there is a buzz — but with so many unknowns, AI quickly feels like a solution in search of a problem. Why waste time and resources trying to develop a solution I cannot implement or that doesn’t address all of my concerns? I asked myself those questions many times in the past as I resisted trying new methods.
In reality, I came to realize this is backward thinking. AI is a powerful tool that can improve credit decision processes. If you have not identified all the problems you need to address and think about how you would apply AI, then implementing an AI strategy for credit decisioning is premature indeed. But, with the right data science partners, you can successfully utilize this new technology to improve your overall risk management practices.
Beyond the buzz
So how do we get past the buzz and actually build tools to reap the benefits of AI in credit decisioning? The first step is creating a true partnership between the data science team and the risk management team. Here’s how you go about doing that:
- Get coffees for everyone
And then right after, kickstart that partnership with an exploratory meeting to discuss, brainstorm, and challenge each other on what the problems are we are trying to solve and how we go about solving them. These stated problems could be broad, such as “I need to reduce delinquency in my credit card portfolio” to more specific such as “I need to know to whom I should market a low-interest auto loan.”
- Dig deep and ask tough questions
The discussion should go deeper to explore all the other possible issues: What data is available for model development? What are my implementation limitations from IT? What are the regulatory issues I have to address? Is there an off-the-shelf AI solution that will work? What is the definition of success?
- Get more coffees (if needed)
Don’t underestimate the amount of time this will take — I have spent entire days in this type of meeting, and it was time well invested. Without a solid understanding of all the problems, the data science team runs the risk of delivering a solution that doesn’t solve the underlying problems. The data science team also has the responsibility to inform the business whether or not AI is the correct solution for the problem at hand.
- Create a tool that predicts good customers, not cat (or dog) people
An AI solution to a problem must be actionable or lead to a concrete decision. Picture your AI team spending weeks analyzing transaction data only to discover they can predict with a strong level of accuracy which of their customers are most likely to own a cat or a dog. This is a fascinating discovery for sure. But I bet you don’t want to be the person having to announce that to your CEO.
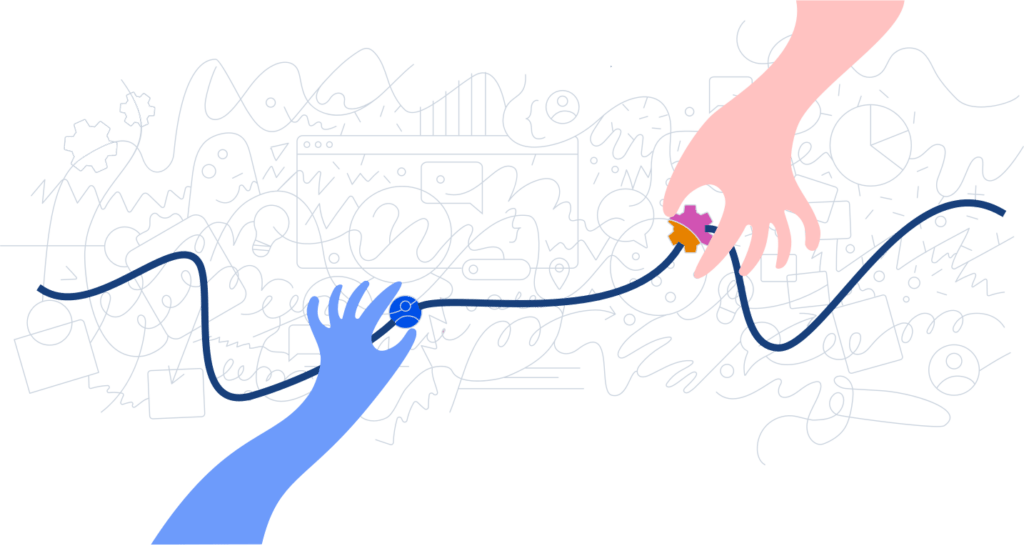
What you need are predictions your teams can take action on and turn into revenue. To kickstart your AI implementation, creating a partnership between your data science and risk management teams is the surest way to identify problems first, and then determine the best tool to solve them.
To go further, go together
The benefits you get from keeping your data scientists in constant contact with their business partners go further than an initial understanding of the problems to solve. Developing an AI tool is not a simple linear process, and you should make sure the data science team can confirm that what they are working on will be used by the business.
Conversely, hearing what the data science team is discovering, the business can think about actions that they have never considered before. Perhaps the data science team will discover a strong relationship between checking account balance trends and the probability that a consumer will default on a loan. This could open the eyes of a risk manager to acquire data they have not traditionally used for decisioning.
And let’s not overlook the fact that sometimes simple solutions will provide most if not all of the information we need. Just because AI is hot, it does not mean that it is the right solution for every situation. Just think of perms in the 80s. As a business, we have to weigh the costs and time associated with using complex techniques versus the return on investment we expect to receive.

Like most data scientists, I love digging into data and using the most advanced techniques possible. It is a fantastic feeling to take a large set of data, apply meaty algorithms, and discover patterns you hadn’t seen before or relationships that you would never have thought of in a million years. However, as exciting as this work is, it is equally if not more disappointing to find out that all the work you did and time you spent will not be used by the business.
In addition to being a practitioner of data science, I have also been a consumer of AI to help me make business decisions. If someone comes to me and says “I can use AI to help your business” without taking the time to understand what my business is and what my problems are, I become skeptical. Are they trying to sell me a tool in search of a problem?