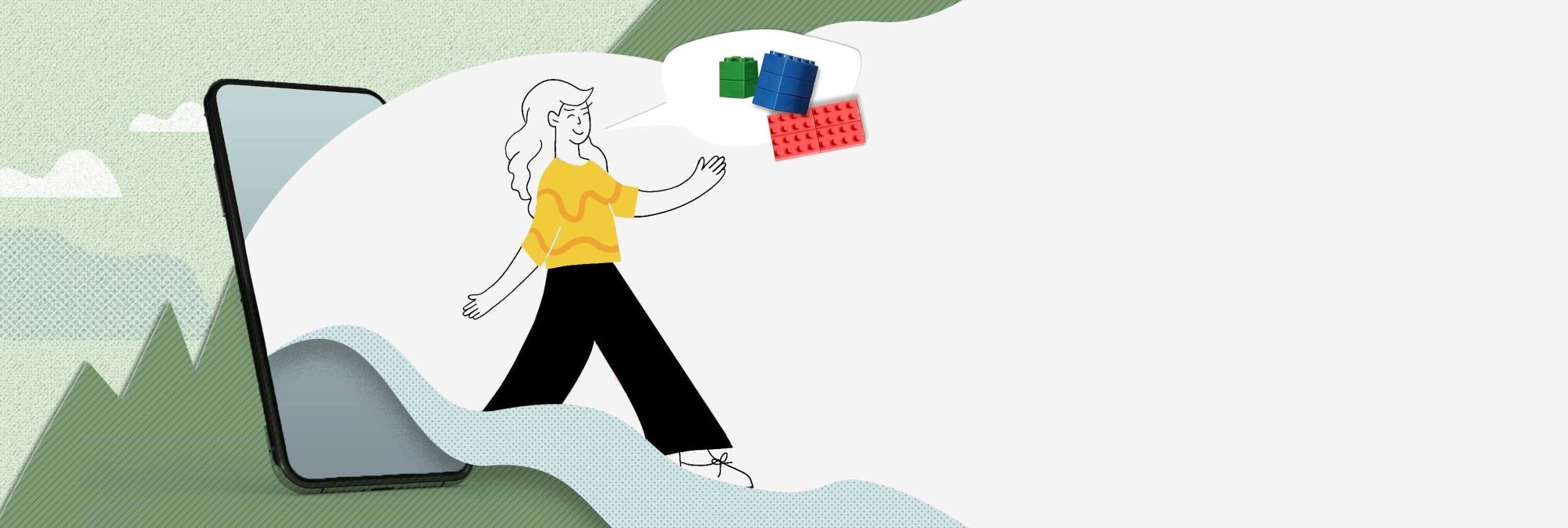
Your business operates on a scale that makes it impossible to intuitively understand the little details that differentiate your customers—and focus on what matters to make your interactions highly meaningful.
There is an inherent tension in growing a business: the focus and resources that allow you to delight your customers might not be sustainable as your customer base gets bigger. You risk delivering a message that feels generic and simply fails to move the needle—which is why companies of all sizes use customer segmentation to target the right audiences with interactions that resonate.
At Flinks, we believe financial data has huge untapped potentials. That's why we built a suite of tools to streamline data collection and gain deep insights on individual customers, so you can deliver more value, faster.
The same data tools you might already be using can also be leveraged to create meaningful engagement, at scale, with groups of customers.
Read on, we’ll walk you through what it means to segment your customers using their transactional data.
Quick links
1. What is customer segmentation?
Customer segmentation is the exercise of grouping customers based on characteristics they have in common. In a very general sense, segmentation allows you to organize information, establish patterns and clarify your customers’ needs so you can take relevant actions.
Marketers use it to send tailored messages and deliver personalized experiences to their ideal customers. Product leaders use it to gain a structured understanding of their users to inform which features to build.
If your organization has sales representatives, segmentation helps focus their efforts by breaking a prospect pool into small groups that can be targeted based on their needs.
Segmenting efficiently drives higher retention rates and customer lifetime value.
A very simple way to represent customer segmentation is through a four-quadrant segment matrix. Each segment shares specific characteristics picked to highlight key differences—this can help identify, for instance, who are currently your best performing customers, or those with the most potential.

In the illustration above, income and free cash flow are used to group customers into four segments. There is no best or worst segment per se—how you interpret your model and determine your key customers really depends on your business goals. For instance, a credit card company might be interested in looking at people with high income and low free cash flow to help them bridge the gap with a special offering.
Common types of data used to segment customers
There are many different data types you can use to segment your customers. The most common are:
- Demographic data
- Geographic data
- Behavioral data
- Psychographic data
In recent years, advances in data processing and analytics have unlocked previously untapped datasets, such as the comprehensive records of your customers’ transactions held by their banks.
A consumer’s transaction history provides direct and indirect data points on all four common types of data used to segment customers.
Thanks to machine learning-powered enrichment tools to categorize it and extract insights, transactional data now provides an incredibly granular perspective on how much a person earns and spends, when and where. A person’s income is a key demographic characteristic, their purchase patterns provide insights on the location of their favorite stores and other customer behaviors, and a deeper analysis will reveal components of their lifestyle.
Segmentation must lead to actions
It is possible—and even advisable, in some contexts—to segment customers using more than one type of data. Regardless of the data type(s) you choose to employ, the concept is the same: segmentation must lead to actions.
To get started, make sure:
- You have access to data.
- Your data is usable to show meaningful differences between your customers.
- You know what you want to segment based upon: geographic location, past transactions, income, life events, the list goes on (and on).
- You have a corresponding action associated with that segmentation. These actions are either predictive or reactive—we’ll see what that can look like in the next section.
Segmentation isn’t an exercise you do once and never revisit.
People change, your products change, even our global context changes—sometimes quite fast. Segmentation is an ongoing effort to understand the meaningful differences that will make your interactions highly relevant to your customers.
2. The value of transactional data for customer segmentation
What actions will be meaningful in your context depends on your business goals in regard to different customer segments, as well as your brand identity and the features of your products.
On the other hand, the type of data you use to segment your customers determines the scope of those actions. How much do you know about your customers? How unique is that information to each individual?
Simply put, the data you use defines your playground. How you decide to play is up to you.
For instance, a person’s transaction history can yield both general data points on how much they earn and spend (high-level insights), or a very granular view of their financial profile and behavior (low-level insights).
In the same vein, a person’s transactional history is a highly individualized footprint. This allows you to focus on a spectrum of things your customers have in common (a macro perspective), down to what uniquely differentiates each of them (a micro perspective).
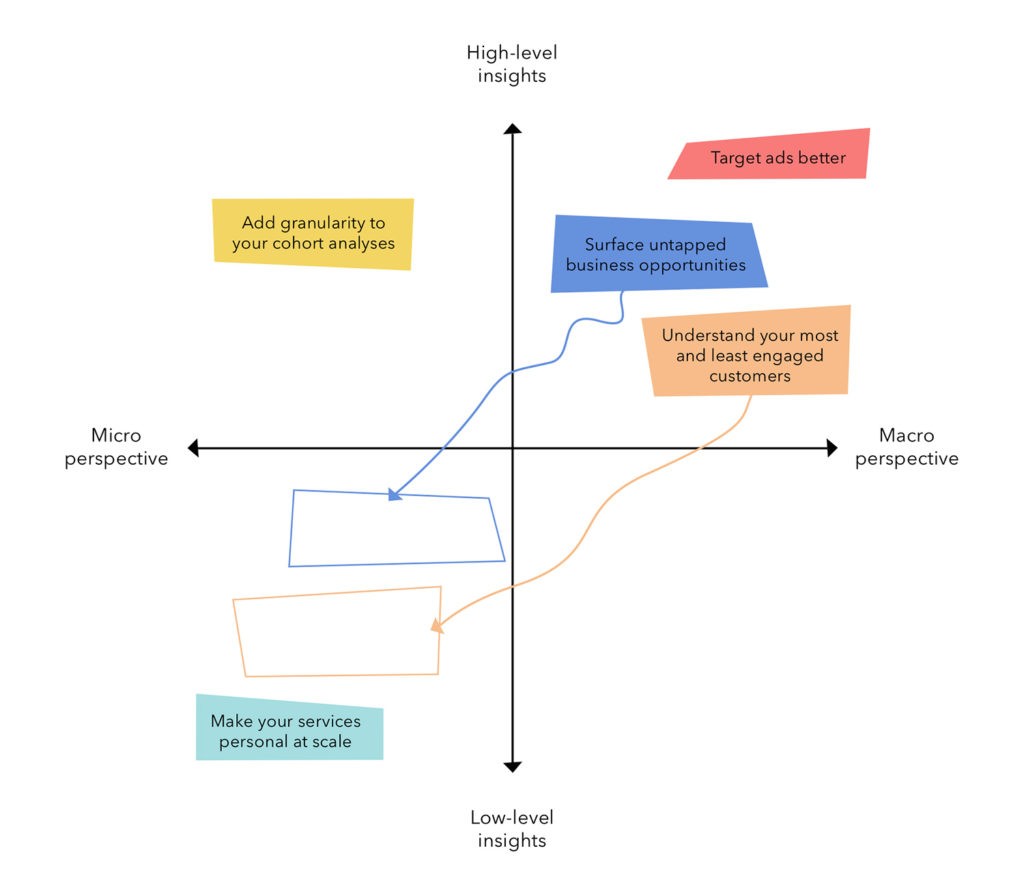
Here’s a short list of use cases for segmenting with transactional data:
Target ads better
Using transactional data in ads targeting means you leverage insights to target blanket differences in your customers—let’s say different income and spending thresholds.
This use case assumes a macro perspective based on high-level insights: you aren’t segmenting based on the individual characteristics of your customers, you’re looking at a couple of factors to determine who should be served your ads.
Add granularity to your cohort analyses
Historically, many firms have had to use very high-level, non-complete or too simple data to segment their cohorts of customers. Using insights, you break the boundary of just using restrictive elements to better view where you're succeeding or not within your business.
This use case assumes a micro perspective based on high-level insights, at least initially: transactional data allows you to use a combination of high-level metrics—income, spend, loan or bill payments, government income, etc.—that are explainable across your business to better understand your clients at the initial high level.
Surface untapped business opportunities
This one is best explained with an example. Let’s say you run a credit card division and you need to find all current clients meeting or exceeding the threshold to get a newly released credit card—income or free cash flow above a specific amount, etc. Your team in charge of segmentation can start by gathering high-level insights for each client to determine which ones are untapped for your product.
Now that you have your pool of potential clients, things start to get interesting. Your team can go deeper and deeper to make that opportunity more real.
Maybe this new credit card offers a special cashback program for bills or utilities payments. Low-level insights from transactional data provide visibility over those specific types of spend for each person within your pool of potential clients, allowing you to segment further. You’re able to create a much more precise, calculated and relevant offer than ever before.
(Whew—and that's just one example.)
To recap: this use case starts with a macro perspective, using high-level insights to identify potential customers. It then shifts to a micro perspective, using low-level insights to narrow down the pool and ensure high relevance.
Understand your most and least engaged customers
This is pretty straightforward. With access to transactional data and insights, you can view patterns within accounts on a macro basis. Combining them allows you to understand what constitutes an engaged or non-engaged customer in the context of your business—this could be usage of the account, ratio of loans you issued to other loans the customer has, etc.
But here’s the thing: being able to identify patterns doesn’t directly lead to understanding what are the right actions to improve engagement engagement rates.
To get there, you need to have a deep view on transactional data. This is done primarily with low-level insights, to isolate what could be the main drivers of (dis)engagement. Transactional data gives you visibility over life events, changes in financial situation, all sorts of purchase and cash flow patterns, and much more—your team will have a field day.
With these elements in hand, you can run experiments across your customer base, and then dive deeper into the individual circumstances and recommended actions that moved the needle in order to refine your hypotheses.
This use case assumes both macro and (mostly) micro perspectives, draws on both high-level and low-level insights. This shows the versatility of transactional data, in that it allows you to tackle engagement as a process of experimentation and optimization.
Make your services personal at scale
Transactional data allows you to gain such a deep understanding of each individual customer that it can in fact be used to create, at scale, the ultimate personalized experience—the segment of one.
This use case assumes a micro perspective based on low-level insights, allowing you to keep track of what makes each customer a unique individual.
3. Next steps
Segmentation is always a part of larger, more complex initiatives. We understand that—it’s why we created tools that make it easy for your team to start leveraging transactional data. If you're already connecting your customers to their bank accounts with Flinks, you're pretty much already set up to feed insights into your segmentation models.
In the next part of this series, we explain three methods to leverage transactional data for customer segmentation: manually, using categorized transactions, and using insights from Attributes.